In this blog post we’re going to discuss Optimizely AB test Statistical Significance, what is the p-value in Optimizely and other more statistical issues in the digital marketing statistics.
Optimizely AB Test Statistical Significance:
Optimizely uses a sophisticated approach to determine the statistical significance of A/B tests. Here are some key points:
- Statistical Significance: This measures how likely it is that your A/B test results are due to the changes you made rather than random chance. Optimizely typically uses a 95% significance level, meaning you can be 95% confident that the results are not due to random chance.
- Sequential Testing and False Discovery Rate Controls: Optimizely’s Stats Engine uses these techniques to calculate significance. This means you don’t need to wait for a pre-set sample size to validate your results.
- Minimum Detectable Effect (MDE): This is the smallest change in conversion rate you want to detect. A smaller MDE requires a larger sample size and longer test duration.
- One-Tailed vs. Two-Tailed Tests: Optimizely uses two-tailed tests to detect differences in both directions (whether your variation is better or worse than the baseline). This is important for controlling the false discovery rate.
- Sample Size Calculator: Optimizely provides a tool to estimate the sample size needed for your tests based on your baseline conversion rate and MDE.
How do you Find the Statistical Significance of an AB Test?
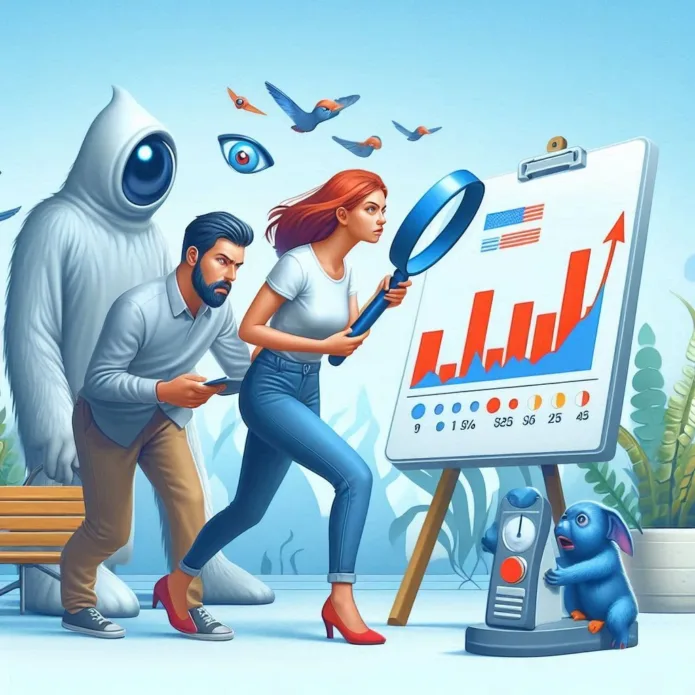
To find the statistical significance of an A/B test, you can follow these steps:
- Formulate Hypotheses:
- Null Hypothesis (H0): Assumes no difference between the two variants.
- Alternative Hypothesis (H1): Assumes a significant difference between the two variants.
- Choose a Significance Level (α):
- Commonly set at 0.05 (5%), meaning there’s a 5% chance of concluding a difference when there isn’t one.
- Collect Data:
- Run your A/B test and collect data on the performance metrics (e.g., conversion rates) for both variants.
- Calculate the Test Statistic:
- Use a formula to calculate the test statistic (e.g., Z-score for large samples or t-score for smaller samples).
- Determine the P-value:
- The p-value indicates the probability of observing the test results under the null hypothesis. A p-value less than α suggests statistical significance.
- Compare P-value with α:
- If the p-value is less than the chosen significance level (α), reject the null hypothesis, indicating a statistically significant difference.
Example Calculation
Let’s say you have the following data:
- Variant A: 1000 visitors, 100 conversions (10% conversion rate)
- Variant B: 1000 visitors, 120 conversions (12% conversion rate)
- Calculate the pooled conversion rate (p): $ p = \frac{100 + 120}{1000 + 1000} = \frac{220}{2000} = 0.11 $
- Calculate the standard error (SE): $ SE = \sqrt{p \cdot (1 – p) \cdot \left(\frac{1}{nA} + \frac{1}{nB}\right)} = \sqrt{0.11 \cdot 0.89 \cdot \left(\frac{1}{1000} + \frac{1}{1000}\right)} \approx 0.014 $
- Calculate the Z-score: $ Z = \frac{pA – pB}{SE} = \frac{0.10 – 0.12}{0.014} \approx -1.43 $
- Find the P-value:
- Use a Z-table to find the p-value corresponding to the Z-score. For Z = -1.43, the p-value is approximately 0.152.
Since the p-value (0.152) is greater than 0.05, you fail to reject the null hypothesis, indicating no statistically significant difference between the variants.
What is the significance level of the AB test?
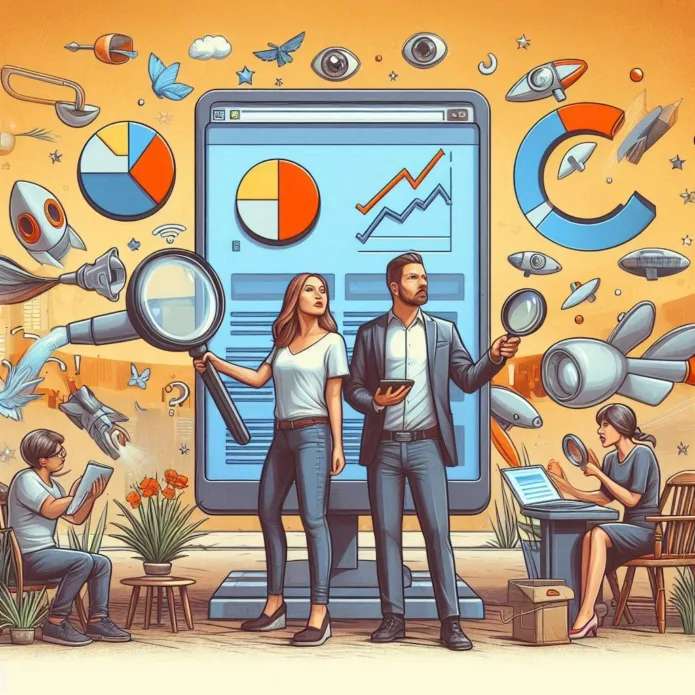
The significance level of an A/B test, often denoted by (\alpha), is the threshold used to determine whether the observed results are statistically significant. It represents the probability of rejecting the null hypothesis when it is actually true (a Type I error).
Commonly, the significance level is set at 0.05 (or 5%). This means there is a 5% chance of concluding that there is a difference between the variants when there is none. In other words, if the p-value of your test is less than 0.05, you would reject the null hypothesis and conclude that the difference between the variants is statistically significant.
Would you like to know more about how to choose an appropriate significance level or how it impacts your test results?
What is the p-value in Optimizely?
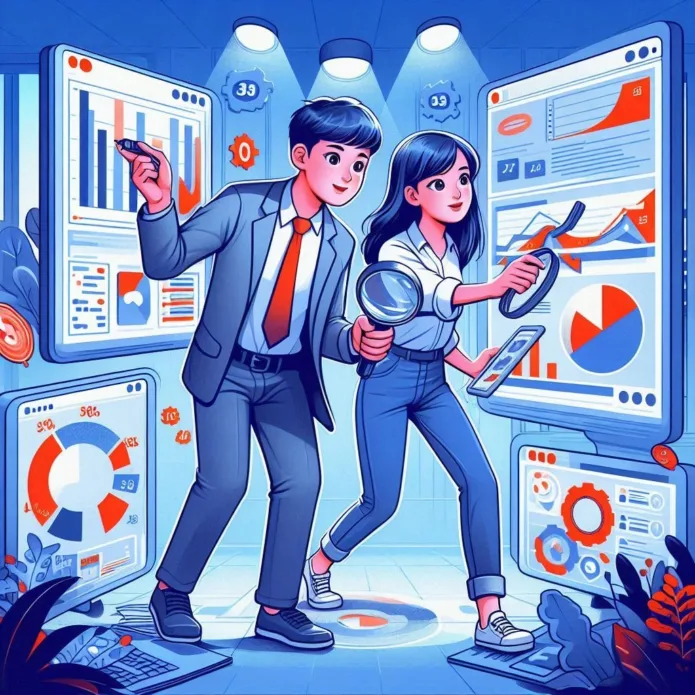
In Optimizely, the p-value represents the probability of observing the test results, or something more extreme, assuming the null hypothesis is true. Essentially, it helps you determine whether the observed differences between your test variations are due to random chance or if they are statistically significant.
Optimizely’s Stats Engine uses a combination of sequential testing and false discovery rate controls to provide p-values that are valid at any point during the test, regardless of sample size. This means you can monitor your results in real-time without worrying about inflating the error rate due to continuous monitoring.
Would you like to know more about how Optimizely’s Stats Engine works or how to interpret p-values in your experiments?
What role does Statistical Significance play in an A B Test?
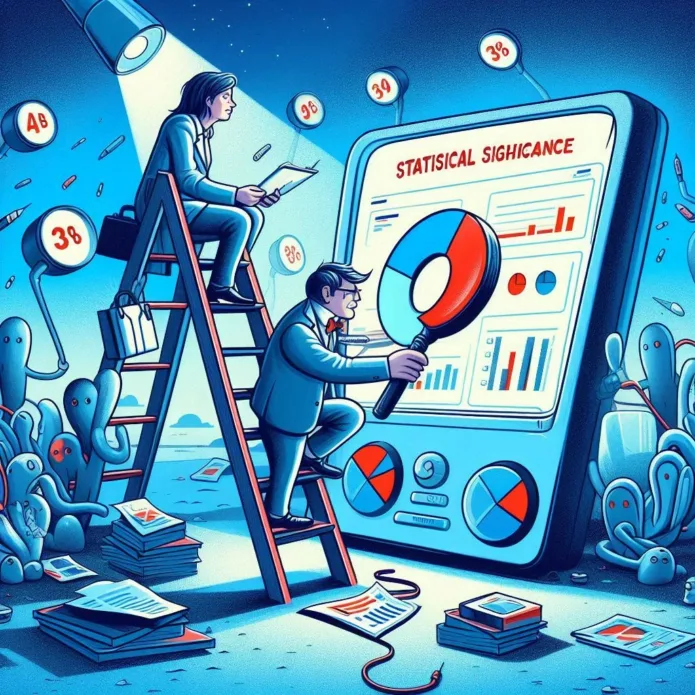
Statistical significance plays a crucial role in A/B testing by helping you determine whether the observed differences between your test variants are meaningful or simply due to random chance. Here are some key points:
- Validation of Results: Statistical significance helps validate that the changes you made in your test are responsible for the observed differences in performance metrics, rather than random fluctuations.
- Decision Making: It provides a basis for making informed decisions. If your test results are statistically significant, you can confidently implement the changes, knowing they are likely to have a real impact.
- Risk Management: By setting a significance level (commonly 0.05), you control the risk of making a Type I error, which is the incorrect rejection of the null hypothesis. This means you reduce the likelihood of concluding that a difference exists when it actually does not.
- Resource Allocation: Ensuring statistical significance helps in efficiently allocating resources. You avoid making changes based on inconclusive or misleading data, which can save time and money in the long run.
- Confidence in Results: Achieving statistical significance increases your confidence that the test results are reliable and can be replicated in future tests or real-world applications.